Archived Content
The National Institute of Mental Health archives materials that are over 4 years old and no longer being updated. The content on this page is provided for historical reference purposes only and may not reflect current knowledge or information.
IRP Podcast: Dr. Laura Lewis: Pushing fMRI into New Realms
Listen
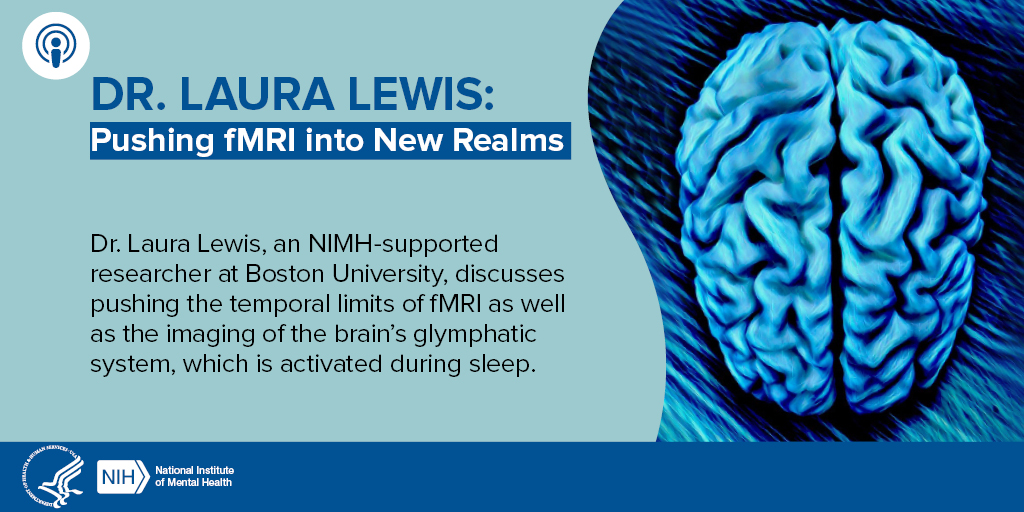
Summary
Dr. Peter Bandettini sits down with Dr. Laura Lewis, an NIMH-supported researcher at Boston University, to discuss her groundbreaking work using fMRI to study the importance of sleep for the brain.
Transcript
Peter Bandettini: Welcome to the Brain Experts podcast, where we meet neuroscience experts and talk about their work, the field in general, and where it's going. We hope to provide both education and inspiration. I'm Peter Bandettini with the National Institute of Mental Health. Please note that the views expressed by the guests do not reflect NIMH policy. This is episode five with Dr. Laura Lewis. Here we discuss pushing the temporal limits of fMRI as well as imaging the brain's glymphatic system that's activated during sleep. Let's chat.
My guest today is Dr. Laura Lewis. She's an assistant professor at Boston University, as well as a former researcher at the Massachusetts General Hospital Martinos Center. She works at the interface between fMRI method development and applications of fMRI to understand rapid brain function dynamics as well as sleep. She's also a rising star in the field of brain imaging. Among her publications is a ground-breaking paper describing how fMRI can extract neuronal oscillations almost as fast as one hertz, and a recent paper in Science describing convincingly the activation of the human glymphatic system during sleep for clearing out brain waste products. Here we talk about her work as well as her background and perspective and pursuing a career in neuroscience research.
So Laura, thank you for joining us on this podcast.
Laura Lewis: Oh, thanks so much for having me.
Peter Bandettini: And I'd just like to start out by just talking a little bit about your background. So you had your undergraduate at McGill University. You got your bachelors in 2008, PhD in neuroscience at MIT under Dr. Emery Brown in 2014. And since then you've just been cranking out some really amazing papers during that time and also then working in collaboration with Mass General Hospital, the Martinos Center. How and when did you first become interested in neuroscience?
Laura Lewis: Yeah. So when I started my undergrad I didn't actually really know that cognitive neuroscience existed. So I was at first a chemistry major and then in my first semester I ended up taking a computer science class - which had not been on my radar - as well as a cognitive neuroscience class. That was the first one I'd had exposure to. I was taught by Michael Petrides at McGill and he had this really cool structure to the class where he basically used a lot of lesion studies where patients have different brain disorders to really kind of help us understand how the structure of the brain is related to the function of the brain. And so I got really excited about both of those things. But this was 2005 and there actually wasn't a neuroscience major in existence yet at the university. So I ended up-- I was very determined to study these two things and I ended up convincing them to let me create my own major, which I just called cognitive science. So that sort of launched me on this path.
Peter Bandettini: And so then you came to MIT and that was with Emery Brown and so how was that? I mean, his focus is mostly on-- at least from what I understand of his research-- is mostly on effects of anesthesia and things like that. So how did that get you started in terms of what you're doing now?
Laura Lewis: I had a really great experience in that lab. And part of what was so cool about it is that he does have this really interesting line of work on understanding states of consciousness and anesthesia and the kind of really systems-level and network-level mechanisms that control arousal states. But the other thread he has running is that he's also a statistician and he has a whole arm of the lab working on signal-processing techniques, and statistical techniques for neuroscience. And so I was coming in more from the background of being interested in these kind of neuroscientific questions about what allows us to kind of be aware in responding to our environment and in the next moment that disappears. But at the same time, I was able to get training and thinking very quantitatively about the signals that we're working with and what's the right kind of analysis or how can we pull new kinds of information out of those signals. And so having that kind of blend of exposure was really useful, and it reflected a kind of unique lab that he runs that really has both of those parts going into it. And anesthesia is an amazing and interesting question, but that's part of what got me thinking more about what I focus on now, which is sleep. And it's something that you don't need a drug. You sleep naturally every single day. And it's clearly something that's essential for the brain. So that's sort of how I ended up turning my attention towards the question of sleep afterwards.
Peter Bandettini: And so like you mentioned, he said he's anesthesia, but he also has quantitative training and sort of combining those disciplines in some sense opens up new fields in some way.
Laura Lewis: Yeah. I think I had really underestimated when I started how essential sort of quantitative and computational approaches are for neuroscience because we have tons of data now and just how to interpret it, I think, is one of the big problems.
Peter Bandettini: So right now, you're working at the interface of fMRI methods and applications. There's a handful of researchers who sort of carved out this niche in some sense between fMRI applications and sort of probing your basic methodology not only in quantitation but also the acquisition and really pushing the methods. What compelled you to sort of move in that direction?
Laura Lewis: Yeah. So I think in my Ph.D., I had been working with invasive recordings in human patients where they actually have electrodes implanted in their brains. And I had also done some studies in animals where we would be able to measure from very deep brain regions. And from all of that, I was getting very excited about the interaction of really large-scaled distributed networks across the brain and key roles for very deep brain structures. And I didn't do any fMRI at that point. But it became clear to me that ultimately I wanted to study the human brain, and I wanted access to these two brain regions. I really needed to move towards fMRI because it has this very kind of special ability to give us these 3-D images of the brain and to include all of these tiny, deep structures that even in animals are actually very difficult to study because if you need to hit a very particular target deep in the brain, that's actually quite challenging to get an electrode there at all.
So I was really kind of motivated to make that change based on the kinds of questions I wanted to ask. And so I only started to learn and work in fMRI in post-doc. And that's when I kind of got excited by realizing how rich the signals are that you can get from fMRI. And particularly, I think there was this opportunity because I was collaborating with these wonderful people at the Martinos Center who had been pushing the temporal resolution. They had ways of getting great new data, and there was this kind of question open to us of like, "Well, could we extract more information from this really fast data to try and get at some of the problems that ultimately I want to answer." So I think it's a really nice intersection because we're very technique limited in neuroscience. And so you could really answer any questions as you push forward the methodology as well. And I also just got really interested in these signals. I mean, they contain so much inside of them, I kind of wanted to know where they were coming from and how we should be analyzing them.
Peter Bandettini: fMRI is still an advancing technique. Yeah. And even people in the field, I think, who use fMRI, I think that they don't always fully appreciate that we're still in the process of trying to figure out how to squeeze more information out of it. There's so much there. At least I believe that there's still a lot there to be extracted if you just do it right.
Laura Lewis: I completely agree. I think there's a ton of information still in there we could go after, and it's actually really fun now to give talks to electrophysiologists and system neuroscientists where I get to kind of be the bearer of good news that there's more we can do and there's kind of doors that are still opening, I think, with this technology. So that's fun.
Peter Bandettini: Yes. And let’s talk a little about your career. So now you just started at Boston University after doing your post-doc and being on the research staff for a while. So how is that transition for the younger scientists who are considering that sort of thing? What was most difficult and most satisfying about it?
Laura Lewis: Yeah. I mean, it's really exciting and really fun and definitely also a big change at the same time. And some of it is just-- any time you move, you have to kind of stop everything and start everything again and lose some months to buy new equipment and just to set up and stuff. It's really exciting to set up a lab. I've always had 10 different projects I wanted to be working on at the same time, and that's not possible when you're just kind of working alone yourself. And it's so great to have trainees looping into the lab and hearing their ideas and being able to establish a broader range of questions we can go after. So that's been really fun. And definitely a challenge in setting up new labs, there's so many sudden new demands on your time, especially when you move to the university setting – there starts to be teaching and committee work and grant writing - so there's many other parts of the job that kind of show up all of a sudden challenging you. And so knowing how to balance all of these kinds of competing things is probably one of the trickier parts of the transition.
Peter Bandettini: So I've been following your work for a while and I think the paper that-- I mean, the paper that first really grabbed my attention and grabbed a lot of people's attention, was your PNAS paper in 2016 on fast fMRI, how can it detect-- or faster fMRI can detect oscillatory neural activity in humans. If you'd like to maybe talk about the main results of that paper, and sort of the implications, that'd be cool.
Laura Lewis: Yeah. Sure. So the way this project started was really coming from this kind of background in sleep and anesthesia. I ultimately really wanted to ask questions about slow oscillations in neural activity in the brain. So these are these oscillatory patterns of brain activity that in humans peak around 0.7 hertz. So a cycle is about a second and a half. And I really wanted to image what was happening in concert with these dynamics because there's lots of evidence suggesting that they're really important for why our kind of neural processing changes so much across brain states. And so I knew that I wanted to integrate EEG and fMRI and a kind of question for us was, would it actually be possible to image brain activity on this timescale with fMRI? And I think the way that a lot of people do their fMRI analysis using kind of standard software packages would suggest no because there's usually this presumption that since fMRI measures kind of the blood's response to neural activity, if that blood response is slow, then we would be measuring slow signals. And so no matter what the neurons are doing, you're kind of limited in how fast you can measure the signal by how fast that kind of hemodynamic blood response is. But as you say, there's kind of all these clues from earlier papers even though in general, people make that assumption, it is also clear from these papers from the '90s that that definitely doesn't always hold. There's, for example, the responses we already knew aren’t linear. We know that if you use really slow stimuli, you get slow responses but if you use faster stimuli, you get faster responses. And there is also your paper which had already kind of shown that as you go up to something like 0.25 hertz, which is already faster than anything that an economical model says you should measure, you were still able to detect that.
Yeah, [crosstalk].
Laura Lewis: And so that's kind of what led to the foundation of this paper is just saying, how fast can we go? And so we wanted to basically control how fast the neurons were changing their activity and check how fast we could keep up with their imaging. And we were imaging pretty rapidly thanks to our collaboration with Kawin Setsompop who had recently developed these accelerated simultaneous, multi-slice techniques for FMRI that perform really well and let us actually get our images much faster than we used to.
And so doing that, we basically used visual stimulation to cause activity in the visual cortex of the brain. And if we stimulate it slowly, we could make the brain patterns slow. If we it stimulate rapidly, we're going to get it fast. And what we found is that we were able to track neuronal activity with FMRI up to about 0.75 hertz. And so that's much faster than was predicted by classic models. And the signals that we saw were about 10 times larger than those predicted by classic models.
And so it's really exciting for us, both in the sense of the fact that this is possible. I think it opens interesting doors for how you could use FMRI. In many of the questions people want to ask in human neuroscience are questions on a scale of hundreds of milliseconds time scale. It's like language processing, complex attentional processes. For me, it's slow oscillations.
Yeah [laughter].
Laura Lewis: You get 0.7 hertz and weirdly right there. Yeah [laughter].
Laura Lewis: It's exciting. It's just that if you scan faster, you really can access much faster information about the brain. And it also kind of then got me interested in where are these fast signals coming from? It's true, many papers have kind of given us signs that this may be possible. But how far can we push it? What's the biology? I think ultimately understanding that will really help us kind of interpret the signals that we're getting as well. So I've been very excited now to use this fast MRI to try and kind of access really time varying dynamics about the brain especially in the context of eat, sleep and wakefulness and altered brain states.
Peter Bandettini: Yeah. And as you say, it hits exactly those frequencies as far as that's concerned. Like you said, it's really-- that's one thing actually also about FMRI. Is that it's sort of surprising and amazing that, you know, everyone models a signal as sort of a linear system. And if it were a linear system, we wouldn't be able to-- we shouldn't be able to see anything.
Laura Lewis: Yeah.
Peter Bandettini: And so it's still kind of a mystery a little bit, as to why we're able to see these oscillations when we drive the signals so fast. Yeah. And do you have any thoughts on that? Why do you think?
Laura Lewis: Yeah. I think there's honestly really several reasons why. I think it's a mix of factors. I think part of it is that the hemodynamic response that we kind of use is true when you look at a large voxel that's kind of averaging across many signals because a lot of the signal comes from large veins that tend to have really big signals and really slow signals. But if you image at high spatial resolution, you can actually see not just fast time to peak but really kind of narrow signals, sort of tighter temporal dispersion.
And so part of it is probably just that when we max these responses originally with kind of slow signals, we kind of weren't paying enough attention to the parts of the brain that have faster ones. So I think part of it is just this kind of higher spatial precision that certain, maybe even the voxels we're more interested in are also the ones that are kind of giving us faster signals. But I think there's also kind of natural nonlinearities. So it seems like a small and rapid change in neural activity can elicit a much faster and larger hemodynamic response than you would have maybe expect from the response that you get to slower to neuron activity.
Peter Bandettini: Yeah.
Laura Lewis: And so I think that nonlinearity is playing into it as well. I think probably both of these things are contributing.
Peter Bandettini: Okay. Yeah. And that sort of plays into to exactly your other paper too, in which you probed this sort of thing. So there's another paper that you have in NeuroImage in 2018, “Stimulus-dependent hemodynamic response timing across the human subcortical-cortical visual pathway identified through high spatiotemporal resolution fMRI 7T.” In that, you sort of just pull out different areas that have different responsivities. And do you want to talk about maybe that a little bit like what causes those differences? And like you were saying, it sort of has something to do with the briefness of the stimulus too that sort of pulls that out.
Laura Lewis: Yeah. So I think I was excited that I could measure these oscillations. But I also felt like I had done it in the easiest scenario. I used the visual cortex which has huge fMRI responses. It's a giant part of the cortex. And as I mentioned before for understanding sleep, we really wanted to understand really tiny deep brain structures like things in the thalamus and the brain stem that are important for understanding sleep. And so we basically wanted to try and do this kind of same mapping in this deeper brain structures and understand are there also fast responses there. We knew from lots of previous great work that the hemodynamic response looks different in different parts of the brain and across different voxels.
And so what we did is we presented a visual stimuli that were either very long or very short, so maybe like 8 seconds or 160 milliseconds, and tried to see how fast the hemodynamic response would be. And we knew from previous work that we would expect it to be faster in response to shorter stimuli and larger in response to shorter stimuli. And what we found is firstly we saw that pattern everywhere we looked. We always saw that these brief stimuli would give you more rapid hemodynamic responses. But we also saw that these responses were faster and faster the deeper we looked in the brain.
So if we looked at a small nucleus of the thalamus, they were almost a second faster, which is a pretty big time difference. And then if we looked into the superior colliculus which is the visual brain stem, we saw that again they were even faster in the thalamus. And so that I think is promising for trying to image fast things in these deep brain regions. The signals are much smaller there, but it's maybe compensated for partly by the fact that the responses are faster there. And then I think it also is just kind of a nice reminder and illustration of how consistent this non-linearity is. And that, in fact, I think it suggests that as people in fMRI move to kind of more naturalistic paradigms where instead of a really long, plain stimulus for eight seconds, maybe they have an actual movie. They have something where contrast levels are fluctuating really rapidly. The hemodynamic response may, in general, be quite a bit faster when there's kind of these more subtle and fast fluctuations in the block case. So I think this might be a kind of more generally applicable model for many of these naturalistic uses too.
Peter Bandettini: Yeah. Yeah. And it opens up-- right. Like you say, I mean, it's sort of like if you continuously stimulate the brain in some way, it opens up sort of this area of high temporal resolution in which you can study interactions a little bit better. So that's great. That's really exciting.
And just to emphasize, there's not a lot of people working on this. So you're really sort of pushing the field in that regard. So that's the one area where you're working. And the other one is your recent paper that has published in Science, it really caught my attention, and it caught a lot of people's attention because, well, the title of it is “Coupled electrophysiological, hemodynamic, and cerebrospinal fluid oscillations in human sleep.” And for myself, ever since there was a first couple of papers a while ago that sort of suggested that there's this lymphatic system that has something to do with sleep, that basically the extracellular fluid is 60% greater during sleep. And then at that point, all the MR physicists sort of said, "Oh, we have to try to find this." And I was looking for it and using diffusion imaging, using T1 changes, T2 changes, whatever. And we haven't yet found something that's super compelling. And so that's why it was so exciting that this really clever idea that you had, taking something that people used and was able to pull out some really interesting results. So if you want to talk about that.
Laura Lewis: Yeah. Sure. Yeah. So I'm excited about this result too. Basically, as you say, over the past few years, there's been a lot of interesting work on this concept of kind of lymphatics in the brain and also a lot of questions about what might the mechanisms be. And I think a lot of this interest is driven around the idea that it's probably very important to remove waste products from the brain. And Mike [inaudible]’s group had shown in a very interesting paper that this seems to happen more during sleep than during wakefulness. There's a higher kind of rate of clearance of things like fluid in the brain when an animal is asleep versus animal is awake.
And as someone who is very interested in sleep, originally for me it was an interest from the question of neural computation. Why do we perceive the world so differently awake versus asleep? But I also started to get really interested in the intersection of brain and body and why is sleep so important for physiological health as well. And so what we ended up doing-- as we were doing this kind of fast fMRI and EEG imaging is realizing that when you do fast fMRI, you also have what's often kind of an artifact in fast fMRI which is that any kind of fluid that's flowing into your imaging volume will be very bright. And what I realized is that we could actually use this to measure the flow of cerebrospinal fluid up into the head. And so cerebrospinal fluid is this liquid that's always enveloping the brain and is important for maintaining homeostasis and kind of removing waste from the brain. And we could actually image how its flow patterns were changing over time. And because we did this with fast fMRI, the imaging we were doing is actually very rapid so we were measuring [inaudible] time scales of hundreds of milliseconds. And I think that was the critical thing that let us see what was happening.
So we have people come in for these midnight studies where they would come in in the middle of the night. We would put an EEG cap on, put them in the scanner, and they would fall asleep. And what jumped out really immediately from these scans is that when people would fall asleep, we would start to see these very large waves of CSF that were happening on this timescale of maybe 1 every 20 seconds or so. And so I think that's part of why it may have been challenging to access through something like diffusion imaging which typically is on a slower timescale than that. But when we were imaging rapidly, you really see-- it's quite striking. You can just sort of see it in the raw images. And so that was already a very exciting finding to us. Just kind of this concept that when people fall asleep, you start to get these very large waves of CSF that seem to be much higher velocity than anything we would on typically see in the awake state. So the first piece was kind of finding those waves at all.
And then the second part was we kind of wanted to understand how they might arise, and we realized that they were very tightly coupled to the [inaudible] signal. So they were most tightly coupled to the temporal derivative of the [inaudible] signal. So you would see whenever the BOLD signal would start to decline, that's when we would get this rush of CSF up into the head. And that suggested to us that what might be happening is as there's less blood in the brain there's kind of more volume available.
More [crosstalk].
And then it's this very simple thing where the CSF would kind of flow in to fill that space. And so the kind of alternation of blood and CSF might be what's happening then during sleep.
Peter Bandettini: so that actually sort of brings to mind the question of the causality. Could it be that the CSF is squeezing out the blood or could be one or the other pushing [crosstalk]?
Laura Lewis: Right. Yeah. That's such an interesting question. So, of course, the study we did was in humans and it doesn't have a causal test to it. So the closest we came to that was doing a computational model of what kind of mechanism would this be consistent with. And the one that we sort of think that this fits with, in the context of the data that we have is that we would find-- first you see a slow wave. So during sleep, there's slow waves or K complexes. There's these events that are kind of large waves in the EEG and they're associated with neurons going quiet for several hundred milliseconds. And we found that those were consistently occurring about six seconds before these CSF waves. So that would be very consistent with a chain of events where the neurons go quiet, they require less blood so, there's less blood flow. The BOLD signal goes down and then where there's less blood volume, there's a CSF flow in. So I think that that is our kind of first bet as to mechanism. But it's an interesting question. I mean it's true. I would expect that if you had something that increased the flow of CSF that that should squeeze the blood vessels. I'm not sure in that case how you would actually get the CSF change. What would drive it?
Peter Bandettini: What causes that. Right. Right.
Laura Lewis: But I would predict, as you say, that if there was another root for driving CSF, then, in turn, that pressure change should also compress the blood vessel.
Peter Bandettini: Yeah. That's interesting. But also, just to make sure it's clear. So BOLD oscillations were-- and that's one thing that's-- so BOLD oscillations are about 0.1 Herz and the CSF oscillations are 0.05 or?
Laura Lewis: No. No. So they're both at 0.05 Herz. Yeah.
Peter Bandettini: They're both at 0.05. Okay. Okay. Just to make sure.
Laura Lewis: Yeah. In the paper we would average across frequency bands, usually from like 0.03 to 0.1 or something, just to kind of not prescribe a very particular peak frequency. But no, their timing was very, was very matched. The BOLD and the CSF were very tightly correlated. Anti-correlated. Yeah.
Peter Bandettini: And it's interesting. It's sort of another clue, it's sort of like-- it's very close to sort of what the breathing frequency is when you're sleeping or whatever. Maybe. Or maybe it's a little faster.
Laura Lewis: So it's quite a bit-- it's slower than breathing.
Peter Bandettini: Or slower than breathing.
Laura Lewis: Yeah. Yeah. So one thing that's interesting is that CSF pulsation is definitely coupled to breathing. And we saw that in wakefulness as well. So CSF is always kind of pulsing a little. And so the fact that it's moving, I think, is not sort of new to us. And there's been a lot of studies showing it's coupling both to breathing and to the heartbeat. And then that makes sense because anything that's kind of moving your tissue around must inevitably move your CSF around as well. And so we saw that in our data too.
But this thing that appeared during sleep was kind of much slower than those dynamics that we've seen with breathing and respiration. But I'm certain that it's going to be coupled to things like respiratory volume as well because when you get these neural events in the EEG, there's often kind of systemic consequences of those. Because when so many neurons change their activity at the same time, then you get kind of downstream effects on lots of other parts of the physiology as well.
Peter Bandettini: Yeah. That's really exciting. I mean, I think that's-- right. This could be a biomarker for people who have differences in oscillations. Might be a biomarker for early Alzheimers or who knows what. I mean, general health or [crosstalk].
Laura Lewis: Yeah. I really hope so. So this study was all-- you know, I get this question. This study was all young, healthy adults, who are ages 20 to 35. Kind of a classic neuroimaging population. But we're doing those follow-up studies now. I would be extremely interested to see how this may change across the lifespan. And particularly with kind of genetic risk for Alzeheimer’s disease. So we're working on that right now. I think it's going to be a really kind of interesting question.
The second area, sleep is really disrupted actually in a number of psychiatric disorders as well and particularly a lot of things associated with neural inflammation. So that's another area where I would really like to follow up and kind of understand how this is related to those. And whether this kind of gives us perhaps a new way to try and track what's happening in the brain.
Peter Bandettini: Yeah. I think it's opened up a lot. Sort of a technical question. So yeah, it's nice that—a lot of people actually look at inflow effects and sort of like an artifact in some sense. But here you're using it. But other methods of MRI can actually-- and I don't know if you looked at this as well, I mean, there is something called phase contrast which-- and there you're not limited to sort of like the edge slices, you can maybe potentially get it all throughout the brain. Have you thought about that or?
Laura Lewis: Yes, actually, so we piloted that.
Peter Bandettini: Okay [laughter].
Laura Lewis: And I think that's going to be something that's going to be really useful to use next. I think this technique was nice for this question because it let us measure the kind of BOLD and CSF at the same time and kind of see this picture and these different kinds of signals interacting. But it had this major limitation that we're measuring CSF flow in one direction only and in one spot only. And so yeah, we've been playing around with the phase contrast imaging to try and kind of get a more 4D picture across multiple regions to understand how do these patterns change in different parts of the brain. That’ll be really important I think for clinical applications as well because in aging just the ventricles expand, and the anatomy is really different, and so we need to be able to look in more than one spot.
Peter Bandettini: Okay. So also in the paper as far as what stage of sleep this is. I think in the popular literature about your paper there was some misunderstanding that it's deep sleep. But do you want to clarify that?
Laura Lewis: Yeah, that would be great. Yeah. So our paper looked specifically at non-rapid eye moment sleep. So the overarching categories of human sleep is that we have rapid eye movement (REM) sleep, which tends to have more vivid dreams, and non-rapid eye movement sleep, which has more of these kinds of slow wave patterns in the EEG. We didn't look at REM sleep at all, so I didn't mean for any of the conclusions to suggest this doesn't happen in REM. I don't know yet. I would be very intrigued to see what's happening to someone during REM. And within non-REM, it can be further broken down into substages of N1, N2, and N3, and that's sort of progressively deep. So N1 is the latest stage of sleep, and N3 is the deepest stage of sleep.
And we didn't break those down really yet in our paper. We included data from all of those, but most of the data is from the intermediate N2 stage. That's actually the stage that we spend the most time in during the night. And so most of the signals that we're measuring were from N2, and I'm going to be very curious in the future to ask is one of these substages the one that has the most of these? And my prediction actually would be that N2 would be the one with the most because you have slow waves, but they're spaced far enough apart from each other that there's time for [inaudible] response. And so that would be my prediction. But we still have to do another study where we get just enough sleep and enough time that we can start to break down these subdivisions between--
Peter Bandettini: Yeah. Which is tricky, right. I mean, so you would do that just simultaneous EEG or in the scanner as well? Which is also technically challenging.
Laura Lewis: Yeah. So to actually sort of say which stage of sleep you're in, you're required to have EEG. And so you need kind of the EEG to electrically define what the stage is, and then need the fMRI to measure the CSF. And then you need the person to actually go into deep sleep inside the scanner, which is a whole other challenge because it's very loud in there. They have to lay on their backs. And so that's, for example, why we didn't have REM sleep in the study. People have to do a full non-REM cycle before they get to REM at the beginning of the night. And so one thing I would like to think about is actually whether there's non-MR ways to get at this as well, where we could get much more natural [inaudible].
Peter Bandettini: Yeah. That's true. Well, there's a lot of work to be done as far as that’s concerned. It's really exciting because it really opens up things. And once again, it reemphasizes the point that there's always-- even after 20, 30 years, there's always new information to be had if we just simply figure out how to look at in a better way.
Going back from that paper, there was one really interesting paper that you wrote in 2012 that you were a part of or that you were first author of, “Rapid Fragmentation of Neural Networks at the Onset of Propofol-induced Unconsciousness.” So propofol is an anesthetic. And this was really interesting. I mean, there's a lot of people who’ve studied sleep, but this was actually intriguing in the sense that it showed a network breakdown. So if you want to talk about that a little bit.
Laura Lewis: Sure, yeah. So I was really interested in what happens as people lose consciousness. And in this case it was very interesting to us to ask this question in the context of general anesthesia. One challenge for us concerning sleep is that as people fall asleep, it's such a gradual and slow process that it's hard to know what's unfolding in a given moment of time. But when people are going in for surgery and given-- typically, they're given a bolus dose of a drug such as propofol. Within seconds someone goes from having a conversation to being completely unaware of their environment. And it's just a totally fascinating transition and also one that's really clinically important to understand for us to kind of know how these drugs are working and what effect they're having on the brain.
So we did this study where we wanted to understand what might be happening in brain networks in anesthesia. What's interesting about these drugs, so Propofol, for example, molecularly, we understand it very well. It's a gaba agonist, it has the effect of kind of enhancing gabaergic inhibition. And so if you took a single cell and you put this drug on it, you would have a strong prediction that maybe the cell just stops firing, right? Maybe there's just less activity. But in the brain, it's much less clear how to make that mapping because what if that cell was an inhibitory cell and it was inhibiting its neighbor. And so kind of taking the mechanism that works at the lower level of one neuron and bringing into what's happening in the network is kind of where we want it to get for understanding, well, what does it really mean for your ability to kind of behave or perform a task?
And so we worked with this very special patient population who really kindly volunteered their time for this study. And it's people who have intractable epilepsy and it hasn't responded to medication. So what they need to do is actually, in this case, go in for surgery to try and remove the part of the brain that's generating seizures. People can really have amazing benefits from this kind of surgery.
And a challenge is that before they do the surgery, they want to know exactly the part of the brain that they're targeting. And so to do that, they've already implanted electrodes in the cortex of the brains of these people so that they can help try and figure out where the seizures were starting.
And so that was the context we were working in. We had these patients with electrodes actually in their brain, some of which were actually measuring single neurons. And then they were going back for surgery to have the electrodes removed and to hopefully kind of have their epilepsy treatment. And it gave us this kind of natural window in which we could look at what's happening to human neurons, and to the human cortex at that moment that they're losing consciousness. And we really wanted to ask what's happening right at that transition.
When you compare being awake to being anesthetized, essentially everything is different. Right? The whole brain is completely different. And so we want to say, "Well, what's happening at the moment where people stop responding to us, right at that transition." And so what we found is that, of course, we know that during anesthesia, neurons fire less, there's less brain activity, but people would lose consciousness a little bit earlier than that. Often, before the neurons start firing-- stop firing at high rates, it's already before that, that somebody has stopped performing a task or stop responding to external stimulus. And what seems to instead happen at the moment that they lose consciousness is we would see this slow oscillatory pattern across the cortex that had very different timing in different brain regions. And so it was as if kind of neurons-- basically neurons would be active for a few hundred milliseconds, and then they would go quiet. And those quiet periods were different in different parts of the brain. And that led us to this concept that essentially, it's as if different parts of the brain were in different time zones. And it was hard for them to ever set up a meeting because if one is in Boston and one in Singapore, even though they're both awake part of the time, it's not at the same time, it's hard to get a connection going.
Peter Bandettini: Yes, yes. So there's not that -- and it seems like that localization you were mentioning that it's sort of down to four millimeters. It seems like the networks, the local correlations are just sort of break off. And there's all these sort of fragmented sort of areas that sort of go up and down, but then that they don't really synchronize.
Laura Lewis: Yeah, exactly. Yeah. So we could sort of see our larger electric grids, things across many centimeters, and you could see this really striking drop off. But things with our sort of smaller electrode within four millimeters of each other did seem to still be quite active at the same time.
Peter Bandettini: It sort of can lead you all the way down a path of sort of understanding consciousness and our conscious experience and things like that, and also just generally understanding, right, what happens during this loss of awareness yeah, this is—And also, once again, it's sort of-- it's nice to have that opportunity to study epilepsy patients, as far as-- with these implanted electrodes, and that otherwise we can never get that.
Laura Lewis: Yeah, it's a really unique data set to have that kind of access. And it's totally fascinating to work with, and directly kind of measure these things. But also very challenging. You can't actually design a bunch of new studies. And so again, that was sort of the pull back to fMRI, where you have a totally non-invasive way to try and measure dynamics. But of course, you can never measure single neurons with fMRI. So, ultimately, it's nice to pull from lots of different techniques to train.
Peter Bandettini: So where do you see in general, see your research going in the future?
Laura Lewis: Yeah. I think that pursuing this path of kind of intertwined thinking about how to use the methods and advance our analyses of the methods in concert with answering these neuroscientific questions is really a productive route. And that's one I hope to pursue. On the method side, there's a couple of things that I would really like to develop that I think will really help us advance fMRI science.
And so one, is how can we better integrate fMRI and EEG signals? They measure very different things. But I think they have so much potential to complement each other. And particularly now that we have fast fMRI, it's really starting to bring it into a timescale that's very kind of relevant for EEG as well. So I think how to fuse these dynamics and try and extract information of those is going to be very interesting, particularly as we move to more and more Seven-Tesla imaging. I think there's all of these incredibly tiny brain structures that we've had very limited access to. And certainly, your work in Laminar fMRI has been really exciting. And I think I'd like to kind of similarly kind of push the spatial resolution for understanding the deep brain structures and their role in modulating fast neurodynamics as we measure in EEG as well.
So figuring out how to get those data processes data, really extract the timing information and extract the underlying [inaudible] dimensional brain states, I think it's going to be a really interesting avenue for us. And ultimately, but I would love to kind of big picture understand is, Why is neural computation so different when you're in a different brain state? So if you just hear a very-- if you hear your alarm going off when you're awake versus when you're asleep versus when you're dreaming, you're going to perceive it so differently and respond so differently. And so how does the intrinsic sort of inner state of the brain shape every computation that it's doing?
I think sleep is a very powerful framework for kind of getting at that bigger question, but that's ultimately what lets us have different emotional states and use context dependence to navigate our environments. So I'd like to kind of get to there to really understand how brain states shape computation. And then ultimately, I think that I'm starting to get excited about the potential of this for clinical applications. And so I really hope that we can also start to use this to understand the many, many conditions where sleep is disrupted, which is many neurological disorders such as Alzheimer's, and also many psychiatric disorders as well have a really significant sleep component to them. And so why is sleep so critical for all of these things the brain does? That's something I'd like to understand.
Peter Bandettini: That's what's so cool, because I mean, there's still questions like that, that are so fundamental. Why do we sleep? And also, like you say, your work is sort of both towards understanding fundamental mechanisms of the brain, but at the same time, and like all the best work in neuroscience and fMRI, it's sort of two-pronged. One, it addresses fundamental questions in the brain and two, there's almost immediate or almost, maybe, a step away or so of clinical applications that relate to it. And it seems like you can go back and forth between this as you push along.
Laura Lewis: Yeah, I'm really excited to sort of inhabit that space of the kind of scientific questions that are-- because it's also all in humans with a lot of imaging that, I hope, have a lot of potential. And it's part of why it's also been so great. All of this work was done with so many fantastic collaborators at the Martinos Center who are thinking about how to advance this imaging as well, so John Polomini, Georgia Vonasar, Chris Rosen, Kawin Setsompop. They've all been developing really exciting techniques that I think have kind of help us translate this now into kind of neuroscientific tools and application.
Peter Bandettini: The last question then: What advice would you give to a new or young potential scientists [inaudible]?
Laura Lewis: That's a big question [laughter]. I mean, there's very granular advice, and very big sort of advice, I guess. And maybe on the granular side, I would say that really looking at and exploring and understanding your data is critical. Because if you want to discover something that you didn't know is in there ahead of time, you have to really see it and kind of poke around and know what's there.
I think the other thing is that writing is so important. And so writing is thinking, and I think it's really useful to kind of actively practice and start writing early and often to try and kind of-- it helps you structure everything you're doing once you're starting to kind of write your plans and think about what conclusions you can reasonably draw from your data.
And also that it's very normal for projects not to work. Most stuff doesn't work [laughter]. And so I think that's often a big shift people have coming from undergrad where the problems were designed to work and then it can be very discouraging when you start research and most things don't work. So I think it's hard but sort of reframing that is kind of, "Okay, well, I learned that that doesn't work," and like, "Let's just try something else." That's a logical part of the process.
Peter Bandettini: All right. I think we've reached the end of our time. And I’d just like to thank you very much for spending the time on this podcast, and I wish you the best of luck in the future. And I look forward to seeing the next papers that come out from your group.