Data Science and Emerging Methodologies in HIV
Overview
The Data Science and Emerging Methodologies program supports research using novel methodological, technological, and data science approaches to advance the use of data to prevent, treat, and ultimately cure HIV. This program supports the development, use, and application of novel assessment tools and digital biomarkers, methodological and data analytic approaches, mathematical and computational methods, and unique data sources, that have the potential to promote the uptake and engagement of HIV testing services, comprehensive HIV treatment and care, and medication and treatment adherence and can identify interventions that are responsive to the complex, interrelated, and dynamic factors associated with HIV. The program also promotes the generation, capture, processing, sharing, and maintenance of DAR-funded data that is findable, accessible, interoperable, and reusable (FAIR), including the use of common data elements, to support ongoing analyses, use, visualization, and communication of high-quality data generated from HIV behavioral and biomedical research.
Areas of Emphasis
- Development of novel approaches for valid and reliable assessments, measures, or estimation of psychological, behavioral, or structural factors that contribute to HIV in cohorts or other large data sources of people living with HIV or high-incidence populations.
- Applications of statistical, mathematical, or computational approaches to integrate and harmonize diverse datasets for the discovery of underlying latent factors in complex behavioral, social, neurological, and biomedical data related to HIV acquisition, care, and comorbidities that may lead to the identification of new intervention strategies.
- Using artificial intelligence and machine learning technologies for understanding and improving HIV prevention and treatment by integrating and harmonizing multimodal (e.g., numerical data, images, text) and multilevel (e.g., individual, community, structural) data collected using various sources such as electronic health records [EHRs], social media, wearables and sensors, smartphones, health care claims data, electronic prescription services, and so forth.
- Scaling data science efforts by generating synthetic datasets to test the effects of HIV prevention and treatment interventions that considers mental health factors as well as social and structural factors on HIV acquisition, engagement in prevention or care, and other HIV outcomes.
Contact
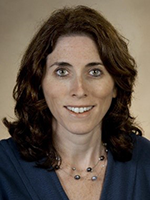
Lori A. J. Scott-Sheldon, PhD
Email: lori.scott-sheldon@nih.gov
Mobile: 301-792-2309
To schedule a meeting: Book time with Scott-Sheldon, Lori (NIH/NIMH) [E]